Data Science: The Blurred Lines between Hype and Reality
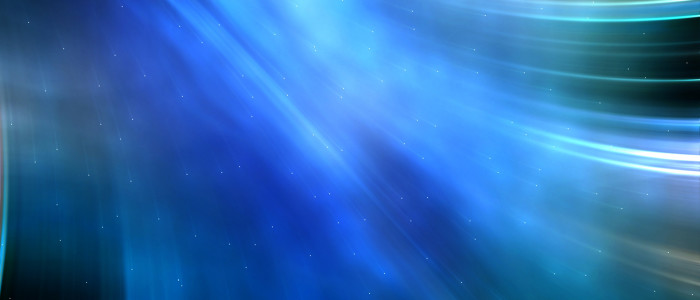
Data Science: The Blurred Lines between Hype and Reality
Over the past years Data Science has experienced a huge surge of popularity in the business world. Whether it is recommending products, making price projections, modeling the behavior of customers or creating self-driving cars, it is not difficult to find examples where Data Science is making a profound impact. Companies such as Google, Microsoft and Amazon are providing cloud services to analyze data and create predictive models. Organizations across the board are embracing the possibilities. With such an increase in popularity and the potential of Data Science applications there is also a huge hype that surrounds it.
As a Data Scientist I firmly believe that we have not even scratched the surface of what data science
can do for businesses. But at the same time, I know how far the field has advanced, which in turn helps
me to easily separate reality from hype. To attain a deep level of understanding in the field usually a
scientific background and a significant time commitment is necessary. Unfortunately this is a luxury
that most decision makers such as CIOs and CTOs don’t have.
In organizations that are heavily relying on Data Science, there are entire teams of Data Scientists
that can readily advise the decision makers on a strategy. However organizations that are implementing
Data Science applications for the first time are in a very different boat. They are more susceptible to
rely on various vendors and to buy into a marketing hype.
For someone without a data science background it is not an easy job to navigate between hype and reality when faced with misinformation. It does not help when there is a shortage of talent and uncertainty in getting good advice. Nor does it help when companies are aggressively hyping up the marketing of their products to make a sale. As a result the line between how far Data Science has advanced and the marketing hype is being blurred.
While decision makers do not have to become experts in Data Science, they should at least be familiar enough with the basics to know when to seek expert advice and how to recognize some of the red flags.
The Science
Data science is about extracting knowledge from data. Usually the underlying science relies heavily on Mathematics, Statistics and Optimization. Approaches in Data Science might originate from Data Mining, Machine Learning, Pattern Recognition, Statistical Modeling or Artificial Intelligence. It encompasses a variety of different sub-fields. In the business world the purpose of Data Science is to learn from past data, find patterns and make predictions that will help guide decision making. This includes also visualizing data and having the ability to design completely new algorithms to answer questions that matter to businesses. Another rather hyped term is Big Data, referring to extremely large data sets. It frequently occurs in conjunction with Data Science, since nowadays most data sets tend to be large. Data Science is however also applicable to smaller data sets and with that also to smaller organizations. You do not have to be a multi-billion dollar company to be able to apply it.
The Hype
The confusion that most decision makers face nowadays is not necessarily about the purpose of Data Science. Most people have heard of high-profile applications such as IBM’s Watson or self-driving cars. Whether the decision involves purchasing a product or initiating a new project the bigger issue is in understanding where the limitations are, and having some sense of what is possible. When being flooded with overblown marketing claims, a general perception that this is the technology of the future, and even prominent personalities such as Bill Gates and Steven Hawking discussing the threat of Artificial Intelligence to the human race, individuals with no background in Data Science can have a hard time distinguishing where the hypothetical ends and reality starts. Hype can come in multiple forms:
- Unsupported claims of superiority. The leading text classification, the most accurate clustering algorithm. No evidence, no explanation whatsoever. The reality might well be that there is entire sea of algorithms that are just as good.
- Exaggeration about what the algorithm does. For example if a neural network is used you might read about how the approach is similar to how the human brain works, along with some fancy illustrations of a digital brain. A sales presentation to individual with no Data Science background might seem like something from a sci-fi movie. Unfortunately it might also raise expectations to sci-fi levels
- Unrepresentative results and use cases. Algorithms tailored to a specific data set and a specific scenario are advertised as broadly applicable solutions.
Unrealistic expectations are generated and the reality of the field is intentionally misrepresented along the way to make a sale. The result is a recipe for failure from a decision-making perspective. Organizations that buy into the hype are turned off from investing any further into Data Science, once reality sets in.
The Reality
The reality is that Data Science is indeed extremely powerful, and can do a lot for your business, In order to ensure success you have to approach it in an informed and strategic manner with realistic expectations in mind. You have to be informed enough to at least know what kind of advice to seek and from whom.
Even though self-driving cars might be around the corner and algorithms exist that can play jeopardy or chess against humans successfully, the underlying science is not advanced yet to be able to learn any arbitrary task you throw at it, as a human being would. The public discussion surrounding the potential threat of Artificial Intelligence is of a philosophical and hypothetical nature, and far away from what is possible today. Data science algorithms are really good at processing large quantities of data, and finding patterns that might not be obvious to the naked eye. However they tend to be always built with a specialized purpose in mind. When developing a self-driving car data scientists have to encode the intelligence the car needs into its models. The car might learn, and adapt to various situations over time, however it will only be able to do that in the context of driving and in the context of its encoded models. The car is not going to be able to generalize its intelligence to start playing chess or perform some completely new task. To make those types of adaptations, Data Scientists have to manually design and implement a new set of models. Who knows what the future will bring, but understanding this process is important when it comes to business applications as well.
Data Science applications in the business world work in a similar way. Data scientists have to design models, which might be adaptive, sophisticated and able to learn over time. If you are working with experienced Data Scientists they will be able to encode knowledge about how your business works into their models, which in turn might lead to more accurate predictions. At the end of the day any time you are tackling a very specialized task which requires specialized industry knowledge, the adaptation is not automatic, instead the underlying predictive models have to be custom-tailored by a Data Scientist to include this domain knowledge.
There is a large collection of freely available tools and algorithms for analysis. You can get started with Data Science without making a huge upfront investment. Before building any tailored models for your business you are well-advised to start out in small steps with algorithms that are available out-of-the-box. This establishes a baseline for performance. Improvements can then be made gradually. This means if you are faced with a proposal which entails waiting for a year before you see any results, you should be careful about committing. Even the most complex projects are more likely to succeed when tackled gradually and in manageable chunks.
Ultimately when contemplating to commit to a vendor of data analysis products or services it is important to get expert advice upfront. A Data Science background is necessary to properly weigh whether claims are founded or overblown, whether there is any scientific credibility. Even if organizations are not willing to share the inner workings of their products, they should be able to provide benchmark comparisons or some evidence supporting their claims. An expert can help weigh the options and determine whether a product is adequate for your needs, and ultimately help you to truly leverage the power of Data Science.