How Predictive Technology is Changing the Game in Maintenance
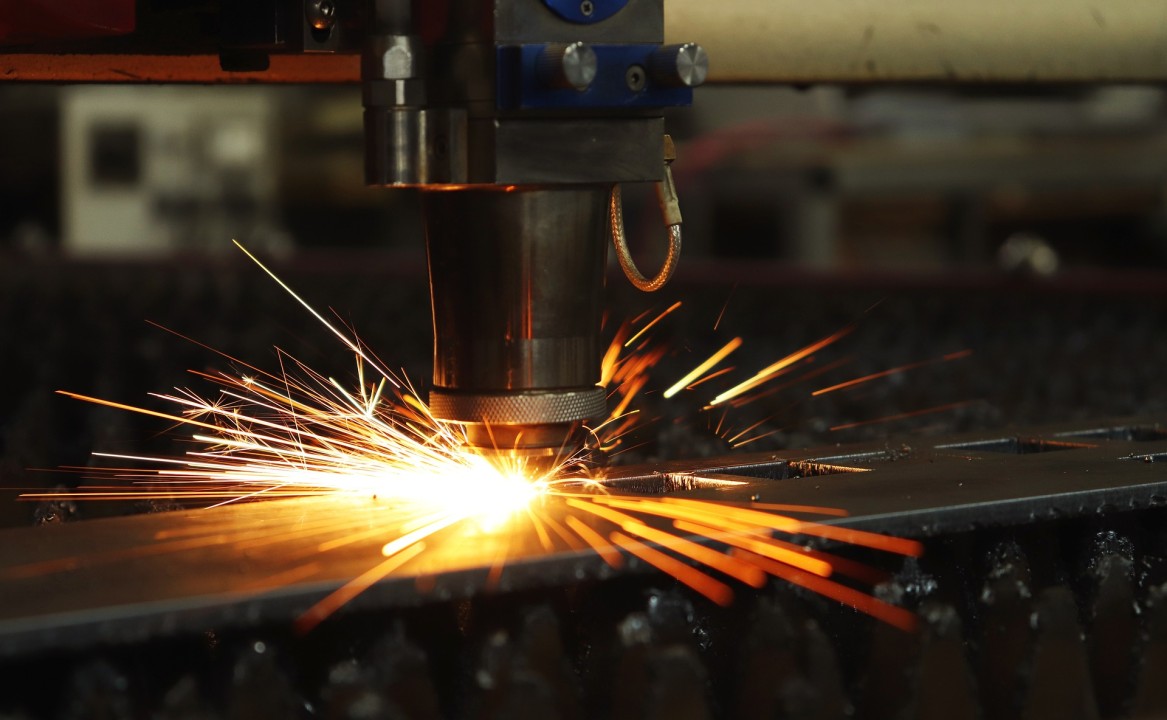
AI and IoT: Unleashing the Power of Digital Twins - Part I
Business Problem
You are a manufacturer. You have a lot of capital tied up in your inventory. Upkeep of that inventory cannot be ad hoc it must be a system. You should be very interested in predictive maintenance. Predictive maintenance is a strategy to optimize your resources tasked with the upkeep. The goal is to be one step ahead in reacting to equipment failure. When successful predictive maintenance indicates when a failure is expected and can even help explain why.
Predictive maintenance is part of preventive maintenance and falls under the broader umbrella of computerized maintenance management. Here we can find additional management functions such as work order, quality control, inventory and so on. It helps to remember that other types of maintenance exist. For example, usage-based preventive maintenance triggers based on utilization. Calendar based maintenance is the most widely used but obviously it is inefficient. As measures of reliability and uptime are concerned, we observe several levels:
-
Reactive: fix when broken
Planned: scheduled or triggered by time
Proactive: triggers on chatter or usage or defect activity
Predictive: triggers on expected reliability
The Value Proposition
The core advantages of predictive maintenance are:
-
Reduced downtime: By identifying potential problems before they occur, businesses can schedule maintenance at a time that is convenient for them, rather than having to respond to unexpected breakdowns.
Improved equipment performance: By identifying and addressing issues that may be causing equipment to perform at less than optimal levels, businesses can improve the efficiency and effectiveness of their equipment, leading to increased production and revenue.
Lower maintenance costs: By identifying issues early on, businesses can take steps to prevent more significant problems from occurring, which can be costly to repair. Additionally, by using data and advanced technologies to optimize maintenance, businesses can reduce the amount of time and resources that need to be allocated to maintenance activities.
Increased safety: Predictive maintenance can help businesses identify potential hazards and take steps to mitigate them before an accident occurs, leading to increased safety for employees, customers, and other stakeholders.
Improved asset utilization: Predictive maintenance can help businesses better understand how their equipment is being used and identify opportunities to improve the utilization of their assets.
Better inventory management: Predictive maintenance allows businesses to predict when they will need to replace certain parts, and can help businesses better manage their inventory, reducing costs and minimizing the risk of stockouts.
Improved customer satisfaction: Predictive maintenance can help businesses improve the uptime and reliability of their equipment, leading to increased customer satisfaction.
Better regulatory compliance: Predictive maintenance can help businesses identify and address issues that may be out of compliance with regulations and guidelines, leading to fewer fines and penalties.
Improved competitiveness: Predictive maintenance can help businesses identify and address issues that may be holding them back from operating at their full potential, leading to improved competitiveness in their market.
Better decision making: Predictive maintenance allows businesses to gather, analyze, and interpret a large amount of data, which can be used to make better, data-driven decisions
Above points are some of the direct consequences of a successful maintenance strategy. Obviously, all of them should lead to improvement in profits and an organization that has a loyal workforce and is robust to turbulences.
Nothing precludes a company from devising a mixed model that would include scheduled, proactive and predictive maintenance. A prediction if reliable should be taken seriously but equally important is the input coming from experience, from a machinery operator.
Challenges in Implementing Predictive Maintenance
Advantages of predictive maintenance are many but not without drawbacks
-
It will cost to acquire predictive software tool stack
It will cost to train or hire staff that can configure tools, analyze data
It will cost to equip your workspace so it can integrate with predictive maintenance
It takes time to implement, technical steps are one, training staff is another
When all is in place: Baseline must be established which means you need to have collected some data
When all is in place: Ongoing monitoring and evaluation will be needed otherwise predictions might drift off
In all the cost is considerable and return on investment could be easily forfeit.
If your company is evolving, it would need to evolve without breaking the data gathering, data analysis and data driven response system that underpins predictive maintenance. That is actually a good thing. Only in a disciplined, well-run company will this be the case. Otherwise, you will pay and will not see better results. Bad results will not be because predictive maintenance is not working but because you are miss-managed and pissing against the wind, so to say.
If your company is not disciplined, you will not take the time to review and adjust and follow through. Failing to do so will give you a system that predicts wrong. Not only will you pay higher maintenance costs, but the people also involved will lose faith. When that happens, they will not react when they should.
It is difficult enough to run a business. Often difficult because of too many moving parts or parameters. Predictive maintenance will constrain some of those parameters which should be a good thing. It will reduce the number of moving parts. It will also create impediments for hasty moves. If a cowboy comes in, makes a change and breaks your predictive maintenance, the cowboy or better yet his disrespect is the problem. Maintenance system relies on timely and exact knowledge about the state of your organization, and it will fail in its mission only if it is blinded by short-sighted heroes!
How AI is Used in Predictive Maintenance
Predictive maintenance uses a variety of artificial intelligence (AI) approaches to monitor the performance of equipment and systems, identify potential issues, and predict when maintenance is needed.
One common AI approach used in predictive maintenance is outlier detection. This involves identifying data points that deviate significantly from the normal range of values, which could indicate potential issues with the equipment or system. Outlier detection can be performed using methods such as statistical analysis, clustering, or rule-based systems. Anomaly detection is a special case of outlier detection, it uses a combination of statistical, rule-based and machine learning methods to identify unusual patterns or behaviors, and detect abnormal events in data streams.
Another AI approach used in predictive maintenance is predictive modeling. This involves using historical data and machine learning algorithms, such as linear regression, decision trees, and neural networks, to predict when maintenance is likely to be needed. Predictive models can be trained using various techniques such as supervised learning, unsupervised learning and reinforcement learning. This can be more effective in detecting failure patterns or patterns of wear and tear that are not necessarily visible as outliers.
The main difference between outlier detection and predictive methods is that outlier detection focuses on identifying data points that deviate significantly from the normal range of values, while predictive methods focus on building models that can make predictions about future events based on historical data.